A Fresh Approach: Opinionated Guide to SDXL Lora Training
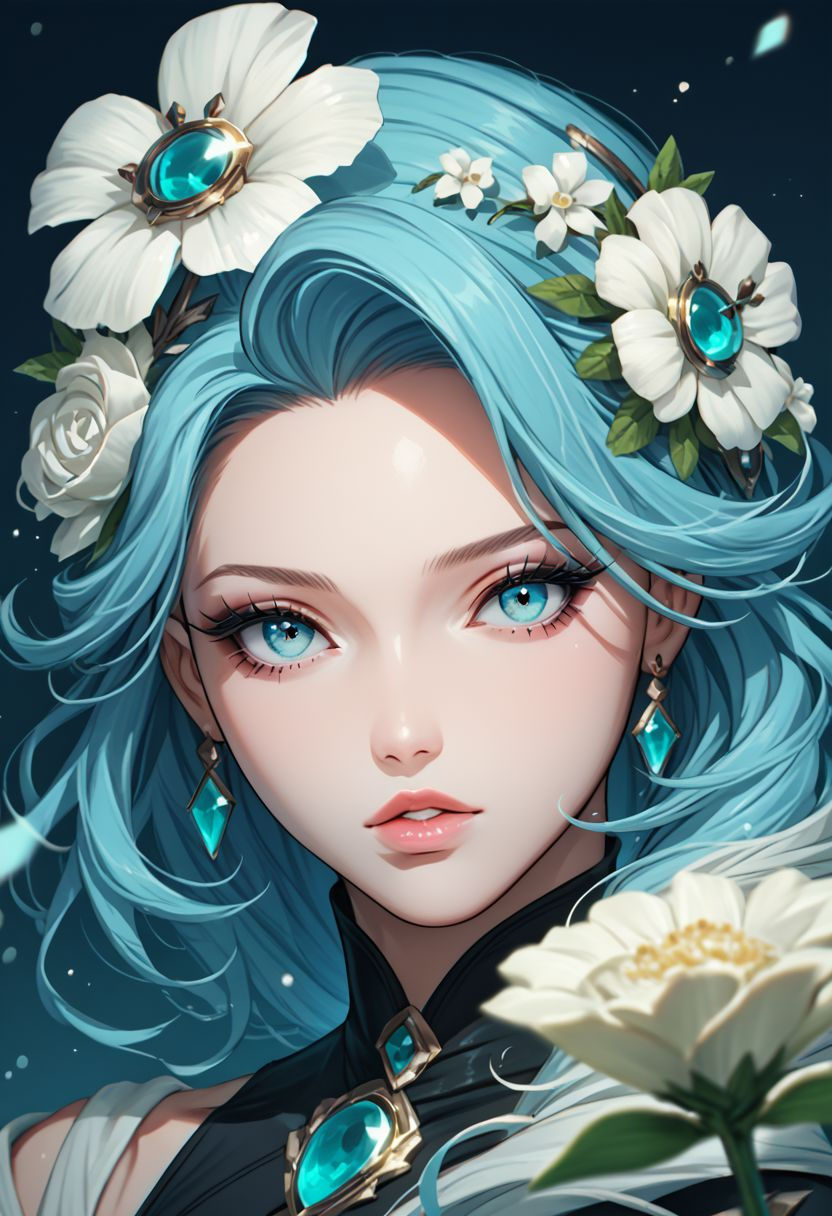
Preface
Welcome to the Fresh Approach version of the Opinionated Guide to SDXL Lora Training!
CivitAi Link to this article here: https://civitai.com/articles/1716/a-fresh-approach-to-sdxl-and-pony-xl-lora-training
Amidst the ongoing discussions surrounding SD3 and model preferences, I'm sharing my latest approach to training SDXL.
I've archived the original article on Ko-Fi and have a version stored on Discord for reference.
The article has been renamed, and more examples plus metadata examples and commentary.
This update features significant rewrites and omits some outdated information, showcasing more recent training methodologies.
Please note, this guide reflects my personal opinions and experiences. If you disagree, that's okay—there's room for varied approaches in this field. There are metadata examples of recent SDXL and Pony trains using Xypher's metadata tool linked below.
A thank you:
Thank you to all of the rest of the Creator Program and Civitai Staff for allowing Earthnicity the space to do what we've been trying to do. Without your continued support, we would not have the strength to keep up with what we're doing.
Original Article was written in August of 2023, as stated above the original SDXL version from 2023 is a PDF we uploaded into Ko-Fi, and the previous version of this is copied into our discord. IF you'd like any information, or the JSON files that were removed as of this update: Please let me know. The Json files are in one of the two backup repositories that are linked at the bottom of this article. Also fixed the support links at the bottom. This version of the article removed a lot of the Metadata examples for clarity, if you'd like to see the metadata examples we've linked back to the Civitai website.
Software Requirements
For offsite training, ensure you have adequate software tools. If your hardware is underpowered (less than 24GB VRAM), consider onsite or cloud-based options for smoother operations.
Software Github & Huggingface Repository Links
(*Local & Server)
I have NOT used all of these and PRE WARNING: Colab's AUP is dodgy AF and may lead to slowdowns. And also not all of these may work as intended. Be careful!
Bmaltais repo: https://github.com/bmaltais/kohya_ss
LastBen: https://github.com/TheLastBen/fast-stable-diffusion
Holo's SDXL for Colab: https://github.com/hollowstrawberry/kohya-colab
Derrian's forked for Colab: https://github.com/Jelosus2/LoRA_Easy_Training_Colab_Frontend
SD Web UI Extension: https://github.com/hako-mikan/sd-webui-traintrain
Image Captioning for ComfyUI: https://github.com/LarryJane491/Image-Captioning-in-ComfyUI
Derrian's back end for SErver: https://github.com/derrian-distro/LoRA_Easy_Training_scripts_Backend
ComfyUI Lora Trainer: https://github.com/LarryJane491/Lora-Training-in-Comfy
Train WebUI: https://github.com/Akegarasu/lora-scripts
OneTrainer: https://github.com/Nerogar/OneTrainer
Lora Studio: https://github.com/michaelringholm/lora-studio
Derrian Main scripts: https://github.com/derrian-distro/LoRA_Easy_Training_Scripts
DOCKER TEMPLATES AS OF 2024 are automatically set up, except in the case where some custom content like Derrian's isn't dockerized:
Runpod: https://runpod.io/?ref=yx1lcptf (our Referral)
VastAI: https://cloud.vast.ai/?ref=70354
Xypher's Lora Metadata: https://xypher7.github.io/lora-metadata-viewer/
Our Tools for Conversion & Backup:
SDXL To Diffusers; https://github.com/duskfallcrew/sdxl-model-converter
Huggingface Backup: https://github.com/duskfallcrew/HuggingFace_Backup
Dataset Editor (in progress WIP): https://github.com/duskfallcrew/Dataset-Tools
Training Essentials for SDXL & Pony XL
First and foremost, quality over quantity in your data collection is crucial. Even a small dataset of around 10 images can yield results. Be mindful of ethical considerations, especially concerning copyrighted content.
Collect Your Data: Sources like Nijijourney, Midjourney, and various online databases can provide diverse image sets. Respect artist rights and consider potential ethical issues.
Upscale as Needed: Aim for images predominantly above 512 pixels, ideally 1024. Onsite, 2048 is the maximum, although consistency is key.
Organize Your Folders: Use structured folder setups like those recommended for BMaltais & Derrian Distro on platforms such as Runpod or local environments. Please note, each of these have their own desired folder setup. Each varies per trainer including One Trainer.
Choosing a Base Model
Offsite & Onsite: I primarily use PonyXL due to personal preference. Animagine is another viable option, despite occasional issues with its text encoder. For broader compatibility, consider SDXL base, which supports most SDXL models except PonyXL. Be aware some of these may cost more if you're using onsite training with buzz.
Repeats and Epochs
The number of repeats depends on your desired level of detail and fidelity in your models. Larger datasets may require adjustments like using Adafactor for PonyXL. (For more information on this please refer to example metadata later on)
Learning Rate and Optimization
2024 Update: Base learning rates are set at 5e4, while text encoder settings for concepts and characters can be adjusted to 1e4. Consider turning off the text encoder for simpler styles.
Optimization: Adafactor and AdamW8Bit are reliable choices, with Prodigy suitable for smaller datasets due to its limitations with larger volumes. However, as of 2024 AdamW8bit as far as i'm aware is not available for SDXL & PonyXL due to mixed information across communities.
Additional Tips
Dataset Organization: Regularly rename files and eliminate duplicates to streamline training. Ensure character-specific datasets remain focused to avoid mixing unrelated elements.
Training Tips: Optimal batch sizes range from 2 to 4 to balance efficiency and quality.
Latents: Always cache latents to enhance training stability and efficiency.
Gradient Checkpointing: Useful for conserving VRAM during training sessions, particularly on lower-spec machines.
Conclusions
SDXL offers advancements over SD 1.5, though hardware compatibility can pose challenges. Remember, training models is akin to navigating nuanced debates—there's no one-size-fits-all solution.
Disclaimer
This guide represents my approach, not definitive rules. Experimentation and adaptation are key to finding what works best for your projects. Removed outdated JSON references and highlighted ongoing updates for clarity and relevance. We have removed the original Civitai article pictures, external links and metadata and if you'd like access to it you may go here: https://civitai.com/articles/1716/a-fresh-approach-to-sdxl-and-pony-xl-lora-training
コメント